Зарегистрируйтесь на getAbstract, чтобы получить доступ к этому краткому изложению.
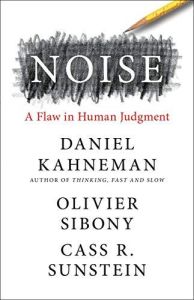
Зарегистрируйтесь на getAbstract, чтобы получить доступ к этому краткому изложению.
Daniel Kahneman, Olivier Sibony and Cass R. Sunstein
Noise
A Flaw in Human Judgment
Little, Brown Spark, 2021
Что внутри?
Wherever judgment exists, you will also find noise – and more of it than you think.
Recommendation
Professor Daniel Kahneman of Thinking, Fast and Slow brings his expertise in decision-making to bear on the phenomenon of noise. When you use your judgment to make evaluations or predictions, you are liable to make errors, without knowing how or why. For instance, people mistakenly believe that errors “cancel each other out” but they don’t. They add up. Examining medicine, the judicial system and insurance, Kahneman and co-authors Olivier Sibony and Cass R. Sunstein expose egregious, undetected errors that a “noise audit” could have diagnosed and avoided. By managing noise, they assert, you can solve problems instead of creating new ones.
Take-Aways
- Physicians, judges, investors and many other professionals show a strikingly high level of disagreement in separate judgments of the same cases.
- “Noise” is variability in judgments that should be identical.
- Noise is found in judgments of the same case by different persons and also in each person’s judgments on separate occasions.
- Noise and bias are independent sources of error.
- Accuracy is always improved when noise is reduced.
- Rules and algorithms are noise-free and often more accurate than humans.
- Breaking up problems and delaying intuition are effective noise-reduction methods
- Eliminating noise entirely is not always a reasonable goal.
Summary
Physicians, judges, investors and many other professionals show a strikingly high level of disagreement in separate judgments of the same cases.
The human mind is a “measuring instrument,” and judgments are the measurements. Therefore, a judgment is a conclusion, not an argument. Making a good judgment is not the same as having good overall judgment. Judgment aims at determining “true value,” which is different for each person. Considerations about judgment include the expectation that people will experience “bounded disagreement.” After all, human beings are fallible – a reflection, in part, of how much judgment varies from person to person.
“A general property of noise is that you can recognize and measure it while knowing nothing about the target or bias.”
Judgments fall into two categories. Inconsistency is problematic in both of them, but for different reasons:
- Predictive judgment – Forecasters judge outcomes on the basis of probabilities. When two doctors or two weather forecasters come to vastly different conclusions using the same data, that indicates noise. Measuring the accuracy of predictive judgments after the fact is almost impossible, especially if the predictions are conditional or long-term. But people still trust their “internal signal of judgment completion,” which they feel enables them to predict an outcome within reasonable bounds.
- Evaluative judgment – These judgments rely on values and preferences, and noise occurs when decisions appear arbitrary, instead of conforming to agreed-on criteria. Disparities in evaluative judgments, particularly in systems supposedly based on evidence, lead to unfairness. Inconsistent judgments tarnish trust and credibility.
“Noise” is variability in judgments that should be identical.
To understand the difference between bias and noise, imagine a target and shooters. Biased shooters consistently miss the bull’s-eye in a recognizable pattern. Noisy shooters produce random scatter, which is more difficult to measure because you cannot tell if the shooters aimed at the target in the first place. Bias indicates consistent deviation from predicted outcomes, such as a scale that consistently adds five pounds to your weight. Noise indicates deviation from an average, such as a manager who consistently underestimates or overestimates how long a project will take. On average, she is right, but the errors add up.
“What people usually claim to strive for in verifiable judgments is a prediction that matches the outcome.”
Noise occurs when conflicting information requires interpretation, and interpreters disagree. Two people may not see a problem in the same light, even if they possess the same knowledge. All they can do is weigh possibilities and assign probability, because there isn’t one clear, correct answer. For example, a candidate for a job may have a difficult character while being ambitious, smart and capable. How do you predict his success as CEO?
In one study, the range among such predictions was from 10% to 95%.How can you calculate error in such a situation in order to avoid future costly mistakes? Carl Friedrich Gauss developed the mean squared error (MSE), which measures the contribution of individual error to overall error. By squaring errors, the MSE places more weight on large ones than small ones. This is central to statistics. While noise and bias are independent, reducing one will likely reduce the other. Multiple regression methodology computes “optimal” weights that minimize squared errors in the original data and can “predict every random fluke.”
Noise is found in judgments of the same case by different persons and also in each person’s judgments on separate occasions.
Since many judgments are predictive and, therefore, verifiable, they teach a lot about noise. A comparison of judgments made by professionals, machines and simple rules finds that professionals commit the most errors, and different professionals commit different errors. To measure this error, a noise audit uses a comparative model called the “percent concordant” to evaluate clinical and mechanical judgments to determine which is more accurate.
For example, take two candidates, and measure how accurately you can predict their eligibility for a job. While a mechanical judgment has more constraints or limits, and weights disparate factors equally, its constraints ensure reliability. Too often, human judgment relies on so many intuitive factors that decision-making becomes almost random. You may think your judgment is more nuanced than a machine’s, but your mood, the moment and your internal preferences can’t replicate the accuracy of a mechanical prediction.
“There is so much noise in judgment that a noise-free model of a judge achieves more accurate predictions than the actual judge does.”
In recent times, machine learning – or AI – has come to prominence in making predictions based on vast troves of data. With greater accuracy than any human, AI is capable of predicting random events. Humans have little tolerance for error in machines, though they tolerate it in themselves. People making predictive judgments too often rely on gut instincts, leading to needless errors.
Wherever prediction exists, ignorance does also – and more than you might think. Admitting ignorance is the first step to addressing uncertainty, and it’s an improvement over allowing overconfidence to flourish and noise to accumulate accordingly.
Noise and bias are independent sources of error.
When people jump to conclusions, they stick to them – either by substituting a simpler question for a difficult one, by prejudging and forcing a conclusion to match their judgment, or by forming coherent impressions quickly and refusing to change them. These three biases contribute to noise. Psychological bias can lead to statistical bias, but everyone has different biases, which create system noise.
“Multiple, conflicting cues create the ambiguity that defines difficult judgment problems.”
When you face difficult, complex or ambiguous decisions, your mind seeks to fulfill two criteria: that your judgment is “comprehensively coherent” and that there isn’t a better alternative. What you believe and think other people believe is not always consistent – for example, because of your mood. These “pattern errors” contribute to pattern noise, which is a combination of stable pattern noise and occasion noise.
Three factors contribute to stable pattern noise: the weight of ranking components, personal reactions and individual qualitative differences among judgments. If you add your unique experiences and your personal quirks, your judgments can be even noisier, though they may be internally consistent in line with your personality.
You can break error down into three successive, layered categories which contribute to noise in different proportions.
- Error divides into bias and system noise.
- System noise divides into level noise and pattern noise.
- Pattern noise divides into stable pattern noise and occasion noise.
Noise contributes more to error than bias does. Among the different kinds of noise, pattern noise is significantly more prevalent than level noise – usually, by at least double.
Accuracy is always improved when noise is reduced.
To improve your judgments, conduct a noise audit by having multiple judges or “decision observers” assess the same problems. The variability in their judgments is noise. If you have a problem with system noise, consider using simple rules or algorithms instead of people. Be aware that AI cannot entirely replace human judgment. Naturally, you want to line up the best judges to improve your error rate, but the factors that make someone a good judge are not always clear. Start with “respect-experts,” people who already have a reputation for good judgment. They will be confident in their judgments and able to explain their reasoning. Because they have many years of experience, they excel at forming coherent narratives.
“Bias leads to errors and unfairness. Noise does too – and yet, we do a lot less about it.”
Alternatively, seek judges whose cognitive style is based on careful thought. These people interrogate information to ascertain whether it is accurate or trustworthy. They are usually humble, as well as more open to criticism and to changing their minds when the facts change. When you are working on a noise audit, these people can observe the decision-making process and alert the team to unidentified biases.
Rules and algorithms are noise-free and often more accurate than humans.
Noise is harder to identify and fix because it is less predictable than bias and harder to explain. To address noise, focus on prevention, not cure. This approach is called “decision hygiene,” and you use it to prevent noise before it happens, like hand-washing among health professionals. You will never know which errors you prevented exactly with frequent hand-washing, but you will have statistically reduced their number.
“Just like hand-washing and other forms of prevention, decision hygiene is invaluable but thankless.”
Some methods for practicing decision hygiene include:
- Sequencing information to limit the formation of premature intuitions – Cognitive bias can affect many professions, such as forensic science. To fix it, give people only the information they need when they need it, and require them to document their judgments at every step.
- Aggregating multiple independent estimates – Forecasting suffers infamous bias, and statistically, forecasters are terrible at their jobs. The easiest fix is to average several judgments since that will dramatically reduce noise.
- Developing diagnostic guidelines – Doctors rely on their training to diagnose disorders, and some are better at it than others. Having guidelines simplifies the process of diagnosis and reduces error.
Breaking up problems and delaying intuition are effective noise-reduction methods.
Everyone dreads performance reviews, which have grown increasingly complex over the years. While they are endemic, they are nonetheless a useless tool for ascertaining an employee’s true worth. To address the noise, create a “shared scale grounded in an outside view,” which cuts down on having too many judgments with too many criteria.
Defining scale in performance ratings is a decision hygiene method. Choose a single dimension, and rank employees compared to one another, rather than using absolute scales. Choose descriptors that are specific enough to be consistent. Ranking can reduce pattern noise and level noise, producing results that are more consistent – and thus, more accurate.
“You can improve judgments by clarifying the rating scale and training people to use it consistently.”
Noise is a problem when you’re hiring new people. Unsurprisingly, interviewers bring cognitive biases to the process. Often, they rely on first impressions, and then seek coherence. The solution? Structure complex judgments by aggregating different judges’ assessments. For example, Google uses these principles in its structure:
- Decomposition – Break the decision down into components. That focuses the judges on the relevant information.
- Independence – Ask predefined questions about candidates’ behavior in various situations.
- Delayed holistic judgment – Do not exclude your intuition about a candidate. Delay it. Form a committee to review all the data interviewers collected to make a collegial decision.
Google is a data-driven company. However, its final decisions are not mechanical, though they are informed by averaging combined scores.
Eliminating noise entirely is not always a reasonable goal.
The costs can outweigh the benefits when you’re trying to eliminate noise. Unfairness is paramount among these costs, since mechanical judgments can’t replace human discernment, particularly when people’s lives are at stake. The financial costs may be too much for public institutions such as educational entities to bear.
Sometimes, noise reduction causes more errors than it fixes. For example, algorithms outperform humans in making noise-free judgments. However, they allow unacceptable biases. Humans value their judgment because it is more discerning and nuanced, and relies on moral underpinnings people want to heed. For instance, mercy is a human quality that no one wants an algorithm to replace or eliminate. If the noise-reduction methods are unfair or crude, but the noise causes irredeemable unfairness, the solution is to create better noise-reduction methods, not to ignore the problem.
“It might be costly to remove noise – but the cost is often worth incurring. Noise can be horribly unfair.”
Social values evolve continuously, and flexibility in judgment can allow new values and beliefs to flourish. In workplaces, having mechanical rules that govern an employee’s tasks can seem dehumanizing, and squelch creativity. However, noise reduction is particularly beneficial in rules-based systems.
Regarding standards – which are more open to interpretation and, therefore, judgment – reducing noise is more desirable. Standards are vague for a reason: They require more nuance. For example, a university may have a standard policy regarding sexual harassment, but not rules for how to behave in every situation. Standards mediate situations in venues where divisions are likely, such as in politics and social situations. Therefore, when you’re exercising judgment, remain aware that your goal is accuracy, not self-expression.
About the Authors
Princeton emeritus professor and 2002 Economic Sciences Nobelist Daniel Kahneman wrote Thinking, Fast and Slow. Former McKinsey senior partner Olivier Sibony teaches strategy at HEC Paris and Saïd Business School, Oxford, and wrote You’re About to Make a Terrible Mistake! Bestsellers by Cass R. Sunstein – Department of Homeland Security senior counselor in the Biden administration and Harvard professor – include How Change Happens, and Nudge, co-authored with Richard Thaler.
This document is restricted to personal use only.
Comment on this summary