Join getAbstract to access the summary!
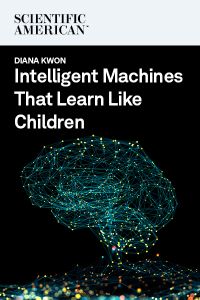
Join getAbstract to access the summary!
Diana Kwon
Intelligent Machines That Learn Like Children
Machines that learn like children provide deep insights into how the mind and body act together to bootstrap knowledge and skills
Scientific American, 2018
What's inside?
Robots that learn like humans and program themselves: Welcome to the brave new world of artificial intelligence.
Recommendation
Artificial intelligence is the decade’s new buzz word. Rapid improvements in computer science and machine learning allow scientists to create robots that have more and more human-like features. One of the newest and most intriguing developments are robots that teach themselves to mimic human development. At the same time these robots allow scientists to better understand the complicated mind and body interactions that bring about human learning, as well as learning deficits. This article will engage and inspire anyone who wants to explore the brave new world of artificial intelligence.
Summary
About the Author
Diana Kwon is a freelance journalist who covers health and the life sciences. She is based in Berlin.
Comment on this summary