Join getAbstract to access the summary!
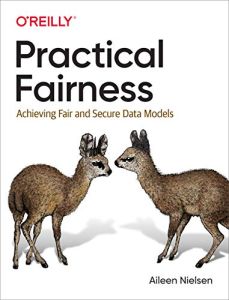
Join getAbstract to access the summary!
Aileen Nielsen
Practical Fairness
Achieving Fair and Secure Data Models
O'Reilly, 2020
What's inside?
Software that learns from data increasingly influences daily life, raising profound questions about its assessments and decisions.
Recommendation
Machine learning is becoming ubiquitous. This branch of artificial intelligence works by teaching a computer program what correct output looks like. This powerful method raises questions regarding fair outcomes for the people machine learning (ML) affects. Software engineer and attorney Aileen Nielsen examines different kinds of fairness and how training data and algorithms can promote them. For those developing machine learning models, she provides useful examples in Python.
Summary
About the Author
Software engineer and lawyer Aileen Nielsen combines work at a deep learning start-up with a fellowship in law and technology at ETH Zürich.
Comment on this summary