Join getAbstract to access the summary!
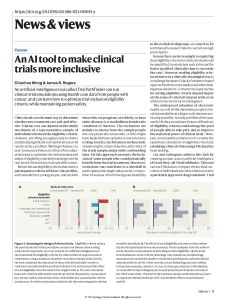
Join getAbstract to access the summary!
Chunhua Weng and James R. Rogers
An AI Tool to Make Clinical Trials More Inclusive
An artificial-intelligence tool called Trial Pathfinder can run clinical-trial emulations using healthcare data from people with cancer, and can learn how to optimize trial-inclusion eligibility criteria, while maintaining patient safety.
Nature, 2021
What's inside?
The AI software Trial Pathfinder optimizes clinical-trial eligibility, while ensuring safety.
Recommendation
The AI software Trial Pathfinder offers hope for medical clinicians looking to safely expand and diversify clinical drug trials, for cancer and other diseases. Chunhua Weng and James Rogers outline a Stanford University research project, led by Ruishan Liu, which revealed the possibility of including people in trials who have often been excluded for reasons of age, health or gender.
Summary
About the Authors
Chunhua Weng and James R. Rogers are members of the Department of Biomedical Informatics at Columbia University.
Comment on this summary