Join getAbstract to access the summary!
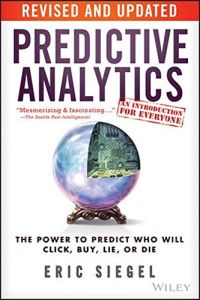
Join getAbstract to access the summary!
Eric Siegel
Predictive Analytics
The Power to Predict Who Will Click, Buy, Lie, or Die
Wiley, 2016
What's inside?
Credit scores were just the start. Big business and big government soon will anticipate your every move.
Recommendation
Predictive analytics (PA) is a concept that’s both undeniably powerful and potentially creepy. This branch of computer science combines big data with statistics to foretell what you might buy, how you might vote or when you might die. Author and predictive analytics guru Eric Siegel is an unabashed cheerleader for his discipline, and he mostly brushes off the privacy concerns it raises. But that’s not to dismiss his study, which is engagingly written and elegantly translates dense materials and abstract concepts into easy-to-read prose. Siegel draws on a number of real-world examples from well-known companies, including Target, Hewlett-Packard and Chase Bank, and he describes his own experience as an expert consultant on predictive analytics. The result is an enlightening, plainly written guide. getAbstract recommends his PA overview to managers and investors seeking insight into a fast-growing corner of the tech economy.
Summary
About the Author
Former Columbia University professor Eric Siegel is the founder of Predictive Analytics World and executive editor of The Predictive Analytics Times.
Comment on this summary